Hello World. Как быть человеком в эпоху машин Фрай Ханна
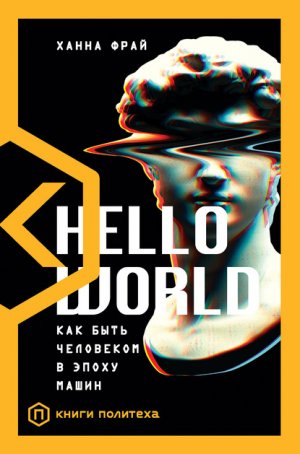
81
John-Paul Ford Rojas, London riots: Lidl water thief jailed for six months, Telegraph, 7 Jan. 2018, http://telegraph.co.uk/news/uknews/crime/8695988/London-riots-Lidl-water-thief-jailed-for-six-months.html.
82
Matthew Taylor, London riots: how a peaceful festival in Brixton turned into a looting free-for-all, Guardian, 8 Aug. 2011, https://theguardian.com/uk/2011/aug/08/london-riots-festival-brixton-looting.
83
Rojas, London riots.
84
Josh Halliday, London riots: how BlackBerry Messenger played a key role, Guardian, 8 Aug. 2011, https://theguardian.com/media/2011/aug/08/london-riots-facebook-twitter-blackberry.
85
David Mills, Paul and Richard Johnson avoid prison over riots, News Shopper, 13 Jan. 2012, http://newsshopper.co.uk/londonriots/9471288. Father_and_son_avoid_prison_over_riots/.
86
Ibid.
87
Rojas, London riots. Ханна Куэрк, специалист по уголовному праву, доцент Манчестерского университета, в 2015 году писала об этом деле: “Как правило, по обвинению такого рода не арестовывают, не запирают в камере и не отдают под суд”. Carly Lightowlers and Hannah Quirk, The 2011 English “riots”: prosecutorial zeal and judicial abandon, British Journal of Criminology, vol. 55, no. 1, 2015, pp. 65–85.
88
Mills, Paul and Richard Johnson avoid prison over riots.
89
William Austin and Thomas A. Williams III, A survey of judges’ responses to simulated legal cases: research note on sentencing disparity, Journal of Criminal Law and Criminology, vol. 68, no. 2, 1977, pp. 306–310.
90
Mandeep K. Dhami and Peter Ayton, Bailing and jailing the fast and frugal way, Journal of Behavioral Decision-making, vol. 14, no. 2, 2001, pp. 141–168, http://onlinelibrary.wiley.com/doi/10.1002/bdm.371/abstract.
91
Почти половина судей разошлись во мнениях о наиболее правильном порядке действий в каждом случае.
92
В статистике есть свой количественный критерий такого согласия в судейских решениях – так называемая каппа Коэна. Метод основан на предположении, что даже при простом угадывании в итоге может быть достигнута сходимость результатов. Полной воспроизводимости соответствует единица. Ноль означает, что оценки хаотичны. Судьи показали баллы от 0 до 1, в среднем 0,69.
93
Diane Machin, Sentencing guidelines around the world, paper prepared for Scottish Sentencing Council), May 2005, https://scottishsentencingcouncil.org.uk/media/1109/paper-31a-sentencing-guidelines-around-the-world.pdf.
94
Ibid.
95
Ibid.
96
Впервые условно-досрочное освобождение начали применять во Франции в 1700-х годах – осужденного выпускали на волю, если он обещал больше не совершать преступлений: https://www.etymonline.com/word/parole.
97
Ernest W. Burgess, Factors determining success or failure on parole, in The Workings of the Intermediate-sentence Law and Parole System in Illinois (Springfield, IL: State Board of Parole, 1928). Эта работа довольно тяжела для восприятия, вместо нее можно почитать статью Кларка Тиббитса, коллеги Бёрджесса, опубликованную позже: Clark Tibbitts, Success or failure on parole can be predicted: a study of the records of 3,000 youths paroled from the Illinois State Reformatory, Journal of Criminal Law and Criminology, vol. 22, no. 1, Spring 1931, https://scholarlycommons.law.northwestern.edu/cgi/viewcontent.cgi?article=2211&context=jclc. Бёрджесс обозначил и другие социальные типы – “белая ворона”, “невольный преступник”, “тупица” и “гангстер”. По его мнению, меньше всех рисковали вновь оказаться на скамье подсудимых люди из категории “деревенщина”.
98
Karl F. Schuessler, Parole prediction: its history and status, Journal of Criminal Law and Criminology, vol. 45, no. 4, 1955, pp. 425–431, https://pdfs.semanticscholar.org/4cd2/31dd25321a0c14a9358a93ebccb6f15d3169.pdf.
99
Ibid.
100
Bernard E. Harcourt, Against Prediction: Profiling, Policing, and Punishing in an Actuarial Age (Chicago and London: University of Chicago Press, 2007), p. 1.
101
Philip Howard, Brian Francis, Keith Soothill and Les Humphreys, OGRS 3: The Revised Offender Group Reconviction Scale, Research Summary 7/09 (London: Ministry of Justice, 2009), https://core.ac.uk/download/pdf/1556521.pdf.
102
Небольшая оговорка: в этой статистике, вероятно, есть систематическая ошибка. Обычно “помощи зала” просят в первых раундах, пока вопросы еще довольно простые. Тем не менее этот феномен, когда коллективное мнение группы оказывается более верным, чем мнение одного человека, находит подтверждение в литературе. См.: James Surowiecki, The Wisdom of Crowds: Why the Many Are Smarter than the Few, New York, Doubleday, 2004, p. 4. Русский перевод: – Шуровьевски Дж. Мудрость толпы. Почему вместе мы умнее, чем поодиночке… М.: “Манн, Иванов и Фербер”, 2014.
103
Netflix Technology Blog, https://medium.com/netflix-techblog/netflix-recommendations-beyond-the-5-stars-part-2-d9b96aa399f5.
104
Shih-ho Cheng, Unboxing the random forest classifier: the threshold distributions, Airbnb Engineering and Data Science, https://medium.com/airbnb-engineering/unboxing-the-random-forest-classifier-the-threshold-distributions-22ea2bb58ea6.
105
Jon Kleinberg, Himabindu Lakkaraju, Jure Leskovec, Jens Ludwig and Sendhil Mullainathan, Human Decisions and Machine Predictions, NBER Working Paper no. 23180 (Cambridge, MA: National Bureau of Economic Research, Feb. 2017), http://nber.org/papers/w23180. На самом деле в этом исследовании использовался “градиентный бустинг над решающими деревьями” – подход, подобный “случайным лесам”, но отличный от них. В обоих методах для принятия решения агрегируются прогнозы множества решающих деревьев, с той разницей, что при градиентном бустинге деревья вырастают последовательно, а в случайных лесах – параллельно. Для этого исследования данные предварительно поделили пополам. На одной половине учили алгоритм, а другую зарезервировали. Готовый к работе алгоритм брал примеры из той половины, которую ему до сих пор не показывали, и пытался предсказать грядущие события. Если бы данные изначально не разделили на две части, алгоритм просто превратился бы в мудреную справочную таблицу.
106
Ученые не пожалели времени на разработку статистических методов для решения этой конкретной задачи, так что все-таки можно провести обоснованное сравнение прогнозов, которые дали люди и машины. Подробнее об этом см. Kleinberg et al., Human Decisions and Machine Predictions.
107
“Costs per place and costs per prisoner by individual prison”, National Offender Management Service Annual Report and Accounts 2015–16, Management Information Addendum, Ministry of Justice information release, 27 Oct. 2016, https://gov.uk/government/uploads/system/uploads/attachment_data/file/563326/costs-per-place-cost-per-prisoner-2015–16.pdf.
108
Marc Santora, City’s annual cost per inmate is $ 168,000, study finds, New York Times, 23 Aug. 2013, http://nytimes.com/2013/08/24/nyregion/citys-annual-cost-per-inmate-is-nearly-168000-study-says.html; Harvard University, Harvard at a glance, https://harvard.edu/about-harvard/harvard-glance.
109
Luke Dormehl, The Formula: How Algorithms Solve All Our Problems… and Create More (London: W. H. Allen, 2014), p. 123.
110
Julia Angwin, Jeff Larson, Surya Mattu and Lauren Kirchner, Machine bias, ProPublica, 23 May 2016, https://propublica.org/article/machine-bias-risk-assessments-in-criminal-sentencing.
111
‘Risk assessment’ questionnaire, https://documentcloud.org/documents/2702103 -Sample-Risk-Assessment-COMPAS-CORE.html.
112
Tim Brennan, William Dieterich and Beate Ehret (Northpointe Institute), Evaluating the predictive validity of the COMPAS risk and needs assessment system, Criminal Justice and Behavior, vol. 36, no. 1, 2009, pp. 21–40, http://northpointeinc.com/files/publications/Criminal-Justice-Behavior-COMPAS.pdf. Согласно исследованию 2018 года алгоритм COMPAS продемонстрировал такую же точность, как “коллектив” респондентов. Исследователи опросили двадцать неподготовленных человек и показали, что их прогноз повторного правонарушения совпадает с оценкой системы COMPAS. Это интересное сравнение, но нельзя забывать, что в реальности у судей нет за дверью бригады незнакомцев, высказывающих свое мнение. Судьи работают в одиночку. И сравнивать им не с чем. См. Julia Dressel and Hany Farid, “The accuracy, fairness, and limits of predicting recidivism”, Science Advances, vol. 4, no. 1, 2018.
113
Christopher Drew Brooks v. Commonwealth, Court of Appeals of Virginia, Memorandum Opinion by Judge Rudolph Bumgardner III, 28 Jan. 2004, https://law.justia.com/cases/virginia/court-of-appeals-unpublished/2004/2540023.html.
114
ACLU brief challenges constitutionality of Virginia’s sex offender risk assessment guidelines, American Civil Liberties Union Virginia, 28 Oct. 2003, https://acluva.org/en/press-releases/aclu-brief-challenges-constitutionality-virginias-sex-offender-risk-assessment.
115
State v. Loomis, Supreme Court of Wisconsin, 13 July 2016, http://caselaw.findlaw.com/wi-supreme-court/1742124.html.
116
Из личной беседы с Ричардом Берком.
117
Angwin et al., Machine bias.
118
Global Study on Homicide 2013 (Vienna: United Nations Office on Drugs and Crime, 2014), http://unodc.org/documents/gsh/pdfs/2014_GLOBAL_HOMICIDE_BOOK_web.pdf.
119
Вероятность такого результата существует всегда, даже без учета пола в расчетах. При любом методе прогнозирования, основанном на таких параметрах, которые в большей мере свойственны одной группе, чем другой – например, на наличии ранее совершенных насильственных преступлений, – несправедливость может иметь место.
120
ACLU, The war on marijuana in black and white, June 2013, https://www.aclu.org/report/report-war-marijuana-black-and-white.
121
Наверное, должно вызвать удивление, что Верховный суд Висконсина поддержал позицию Equivant. Эрик Лумис, которого судья при помощи оценочного инструмента COMPAS приговорил к шести годам тюремного заключения, подал апелляцию. В деле “Лумис против штата Висконсин” говорилось, что использование находящейся в частной собственности компьютерной программы с закрытым кодом для оценки риска и вынесения приговора нарушало право обвиняемого на справедливое рассмотрение дела, так как защита не могла оспорить научную обоснованность оценки. Однако Верховный суд Висконсина постановил, что применение судом первой инстанции машинного средства оценки при вынесении приговора не нарушало право подсудимого на надлежащую правовую процедуру: Supreme Court of Wisconsin, case no. 2015AP157-CR, opinion filed 13 July 2016, https://wicourts.gov/sc/opinion/DisplayDocument.pdf?content=pdf&seqNo=171690.
122
Lucy Ward, Why are there so few female maths professors in universities? Guardian, 11 March 2013, https://theguardian.com/lifeandstyle/the-womens-blog-with-jane-martinson/2013/mar/11/women-maths-professors-uk-universities.
123
Sonja B. Starr and M. Marit Rehavi, Racial Disparity in Federal Criminal Charging and Its Sentencing Consequences, Program in Law and Economics Working Paper no. 12–002 (Ann Arbor: University of Michigan Law School, 7 May 2012), http://economics.ubc.ca/files/2013/05/pdf_paper_marit-rehavi-racial-disparity-federal-criminal.pdf.
124
David Arnold, Will Dobbie and Crystal S. Yang, Racial Bias in Bail Decisions, NBER Working Paper no. 23421 (Cambridge, MA: National Bureau of Economic Research, 2017), https://princeton.edu/~wdobbie/files/racialbias.pdf.
125
John J. Donohue III, Capital Punishment in Connecticut, 1973–2007: A Comprehensive Evaluation from 4686 Murders to One Execution (Stanford, CA, and Cambridge, MA: Stanford Law School and National Bureau of Economic Research, Oct. 2011), https://law.stanford.edu/wp-content/uploads/sites/default/files/publication/259986/doc/slspublic/fulltext.pdf.
126
Adam Benforado, Unfair: The New Science of Criminal Injustice (New York: Crown, 2015), p. 197.
127
Sonja B. Starr, Estimating Gender Disparities in Federal Criminal Cases, University of Michigan Law and Economics Research Paper no. 12–018 (Ann Arbor: University of Michigan Law School, 29 Aug. 2012), https://ssrn.com/abstract=2144002 или http://dx.doi.org/10.2139/ssrn.2144002.
128
David B. Mustard, Racial, ethnic, and gender disparities in sentencing: evidence from the US federal courts, Journal of Law and Economics, vol. 44, no. 2, April 2001, pp. 285–314, http://people.terry.uga.edu/mustard/sentencing.pdf.
129
Daniel Kahneman, Thinking, Fast and Slow (New York: Farrar, Straus & Giroux, 2011), p. 44. Русское издание: Канеман Д. Думай медленно… решай быстро. М.: АСТ, 2017.
130
Если бы мяч стоил 10 пенсов, то бита должна была бы стоить 1 фунт 10 пенсов, что в сумме составило бы 1 фунт 20 пенсов.
131
Chris Guthrie, Jeffrey J. Rachlinski and Andrew J. Wistrich, Blinking on the Bench: How Judges Decide Cases, paper no. 917 (New York: Cornell University Law Faculty, 2007), http://scholarship.law.cornell.edu/facpub/917.
132
Kahneman, Thinking, Fast and Slow, p. 13.
133
Ibid., p. 415.
134
Dhami and Ayton, Bailing and jailing the fast and frugal way.
135
Brian Wansink, Robert J. Kent and Stephen J. Hoch, An anchoring and adjustment model of purchase quantity decisions, Journal of Marketing Research, vol. 35, 1998, pp. 71–81, http://foodpsychology.cornell.edu/sites/default/files/unmanaged_files/Anchoring-JMR-1998.pdf.
136
Mollie Marti and Roselle Wissler, Be careful what you ask for: the effect of anchors on personal injury damages awards, Journal of Experimental Psychology: Applied, vol. 6, no. 2, 2000, pp. 91–103.
137
Birte Englich and Thomas Mussweiler, Sentencing under uncertainty: anchoring effects in the courtroom, Journal of Applied Social Psychology, vol. 31, no. 7, 2001, pp. 1535–1551, http://onlinelibrary.wiley.com/doi/10.1111/j.1559–1816.2001.tb02687.x.
138
Birte Englich, Thomas Mussweiler and Fritz Strack, Playing dice with criminal sentences: the influence of irrelevant anchors on experts’ judicial decision making, Personality and Social Psychology Bulletin, vol. 32, 2006, pp. 188–200, https://researchgate.net/publication/7389517_Playing_Dice_With_Criminal_Sentences_The_Influence_of_Irrelevant_Anchors_on_Experts%27_Judicial_Decision_Making. Criminal sentencing by preferred numbers, Journal of Empirical Legal Studies, vol. 17, no. 1, 2020, pp. 139–163. https://doi.org/10.1111/jels.12246.
139
Ibid.
140
Ibid.
141
Mandeep K. Dhami, Ian K. Belton, Elizabeth Merrall, Andrew McGrath and Sheila Bird, Sentencing in doses: is individualized justice a myth? (готовится к печати). С любезного разрешения Мандип Дхами, из личной беседы с ней.
142
Ibid.
143
Adam N. Glynn and Maya Sen, Identifying judicial empathy: does having daughters cause judges to rule for women’s issues? American Journal of Political Science, vol. 59, no. 1, 2015, pp. 37–54, https://scholar.harvard.edu/files/msen/files/daughters.pdf.
144
Shai Danziger, Jonathan Levav and Liora Avnaim-Pesso, Extraneous factors in judicial decisions, Proceedings of the National Academy of Sciences of the United States of America, vol. 108, no. 17, 2011, pp. 6889–6892, http://pnas.org/content/108/17/6889.
145
Keren Weinshall-Margel and John Shapard, Overlooked factors in the analysis of parole decisions, Proceedings of the National Academy of Sciences of the United States of America, vol. 108, no. 42, 2011, E833, http://pnas.org/content/108/42/E833.long.
146
Uri Simonsohn and Francesca Gino, Daily horizons: evidence of narrow bracketing in judgment from 9,000 MBA-admission interviews, Psychological Science, vol. 24, no. 2, 2013, pp. 219–224, https://ssrn.com/abstract=2070623.
147
Lawrence E. Williams and John A. Bargh, Experiencing physical warmth promotes interpersonal warmth, Science, vol. 322, no. 5901, pp. 606–607, https://ncbi.nlm.nih.gov/pmc/articles/PMC2737341/.
148
Richard M. Levenson, Elizabeth A. Krupinski, Victor M. Navarro and Edward A. Wasserman. Pigeons (Columba livia) as trainable observers of pathology and radiology breast cancer images, PLOSOne, 18 Nov. 2015, http://journals.plos.org/plosone/article?id=10.1371/journal.pone.0141357.
149
Hippocrates’ daughter as a dragon kills a knight, in “The Travels of Sir John Mandeville”, British Library Online Gallery, 26 March 2009, http://bl.uk/onlinegallery/onlineex/illmanus/harlmanucoll/h/011hrl000003954u00008v00.html.
150
Eleni Tsiompanou, Hippocrates: timeless still, JLL Bulletin: Commentaries on the History of Treatment Evaluation (Oxford and Edinburgh: James Lind Library, 2012), http://jameslindlibrary.org/articles/hippocrates-timeless-still/.
151
David K. Osborne, Hippocrates: father of medicine, GreekMedicine.net, 2015, http://greekmedicine.net/whos_who/Hippocrates.html.
152
Richard Colgan, Is there room for art in evidence-based medicine? AMA Journal of Ethics, Virtual Mentor 13: 1, Jan. 2011, pp. 52–54, http://journalofethics.ama-assn.org/2011/01/msoc1–1101.html.
153
Joseph Needham, Science and Civilization in China, vol. 6, Biology and Biological Technology, part VI, Medicine, ed. Nathan Sivin (Cambridge: Cambridge University Press, 2004), p. 143, https://monoskop.org/images/1/16/Needham_Joseph_Science_and_Civilisation_in_China_Vol_6–6_Biology_and_Biological_Technology_Medicine.pdf.
154
“Ignaz Semmelweis”, Brought to Life: Exploring the History of Medicine (London: Science Museum n.d.), http://broughttolife.sciencemuseum.org.uk/broughttolife/people/ignazsemmelweis.
155
“Где Уолли?” – серия детских книг Мартина Хендфорда, где нужно найти человечка Уолли на картинках среди множества людей. (Прим. ред.)
156
Из личной беседы с Энди Беком.
157
Joann G. Elmore, Gary M. Longton, Patricia A. Carney, Berta M. Geller, Tracy Onega, Anna N. A. Tosteson, Heidi D. Nelson, Margaret S. Pepe, Kimberly H. Allison, Stuart J. Schnitt, Frances P. O’Malley and Donald L. Weaver, Diagnostic concordance among pathologists interpreting breast biopsy specimens, Journal of the American Medical Association, vol. 313, no. 11, 17 March 2015, 1122–32, https://jamanetwork.com/journals/jama/fullarticle/2203798.
158
Ibid.
159
Термин “нейросеть” взят по аналогии с мозговыми процессами. В мозге миллиарды нейронов связываются друг с другом, образуя колоссальную сеть. Каждый нейрон слушает своих соседей и, получив сигнал от другого возбужденного нейрона, переправляет его дальше. Сигнал возбуждает следующие нейроны, которые его слушают.
Нейросеть – это сильно упрощенная и более упорядоченная версия мозга. Ее искусственные нейроны располагаются слоями, все нейроны каждого слоя слушают нейроны предыдущего слоя. В нашей задаче с собакой отдельные пиксели изображения – это первый слой. За ним идут несколько слоев с тысячами нейронов в каждом, последний слой представляет собой единственный нейрон, который выдает вероятность того, что на исходной картинке изображена собака.
Процедура корректировки нейронов называется алгоритмом обратного распространения ошибки. Все начинается с последнего нейрона, оценивающего вероятность того, что в программу ввели изображение собаки, а не кого-то или чего-то еще. Допустим, мы ввели изображение собаки и получили предположение, что с вероятностью 70 % на картинке изображена собака. Нейрон воспринимает сигналы, поступившие с предыдущего слоя, и сообщает: “В следующий раз, когда я получу такую информацию, я увеличу вероятность того, что на изображении есть собака”. Затем он обращается к каждому из нейронов предыдущего слоя: “Привет, если вы вместо этого сигнала дадите мне такой-то, я отвечу поточнее”. Все эти нейроны смотрят на свои входящие сигналы и корректируют исходящие для следующей попытки. Далее они сообщают предыдущему слою, какие сигналы те должны послать в свою очередь – и так далее, слой за слоем, вплоть до самого первого. От этого процесса передачи сообщений об ошибке по всей нейросети назад и происходит термин “обратное распространение ошибки”.
Подробнее о нейросетях – об их устройстве и обучении – можно почитать в книге Педро Домингоса “Верховный алгоритм: как машинное обучение изменит наш мир”. (русский перевод В. Горохова, М.: “Манн, Иванов и Фербер”, 2016).
160
Alex Krizhevsky, Ilya Sutskever and Geoffrey E. Hinton, ImageNet classification with deep convolutional neural networks, in F. Pereira, C. J. C. Burges, L. Bottou and K. Q. Weinberger, eds, Advances in Neural Information Processing Systems 25 (La Jolla, CA, Neural Information Processing Systems Foundation, 2012), pp. 1097–1105, http://papers.nips.cc/paper/4824-imagenet-classification-with-deep-convolutional-neural-networks.pdf. Этот алгоритм получил название “свёрточная нейронная сеть”. Для него не загружают исходное изображение полностью – сначала эта программа применяет множество различных фильтров и ищет в измененном изображении включенные в него небольшие паттерны.
161
Marco Tulio Ribeiro, Sameer Singh and Carlos Guestrin, “Why should I trust you?” Explaining the predictions of any classifier, 22nd ACM SIGKDD International Conference on Knowledge Discovery and Data Mining, San Francisco, 2016, pp. 1135–1144, http://kdd.org/kdd2016/papers/files/rfp0573-ribeiroA.pdf.
162
Ответы докторов сравнивали с коллективными результатами анализа проб, выполненного группой экспертов, – их заключение принималось за “эталон”.
163
Trafton Drew, Melissa L. H. Vo and Jeremy M. Wolfe, The invisible gorilla strikes again: sustained inattentional blindness in expert observers, Psychological Science, vol. 24, no. 9, Sept. 2013, pp. 1848–1853, https://ncbi.nlm.nih.gov/pmc/articles/PMC3964612/.
164
Горилла находится вверху справа.
165
Yun Liu, Krishna Gadepalli, Mohammad Norouzi, George E. Dahl, Timo Kohlberger, Aleksey Boyko, Subhashini Venugopalan, Aleksei Timofeev, Philip Q. Nelson, Greg S. Corrado, Jason D. Hipp, Lily Peng and Martin C. Stumpe, Detecting cancer metastases on gigapixel pathology images, Cornell University Library, 8 March 2017, https://arxiv.org/abs/1703.02442.
166
Dayong Wang, Aditya Khosla, Rishab Gargeya, Humayun Irshad and Andrew H. Beck, Deep learning for identifying metastatic breast cancer, Cornell University Library, 18 June 2016, https://arxiv.org/abs/1606.05718.
167
David A. Snowdon, The Nun Study, Boletin de LAZOS de la Asociacin Alzheimer de Monterrey, vol. 4, no. 22, 2000; D. A. Snowdon, Healthy aging and dementia: findings from the Nun Study, Annals of Internal Medicine, vol. 139, no. 5, Sept. 2003, pp. 450–454.
168
Плотность мыслей как индикатор лингвистической развитости рассчитывалась по количеству отдельных мыслей монахини на фразу из десяти слов. Об этом есть интересная статья: Associated Press, Study of nuns links early verbal skills to Alzheimer’s, Los Angeles Times, 21 Feb. 1996, http://articles.latimes.com/1996-02-21/news/mn-38356_1_alzheimer-nuns-studied.
169
Maja Nielsen, Jrn Jensen and Johan Andersen, Pre-cancerous and cancerous breast lesions during lifetime and at autopsy: a study of 83 women, Cancer, vol. 54, no. 4, 1984, pp. 612–615, http://onlinelibrary.wiley.com/wol1/doi/10.1002/1097–0142 (1984) 54:4 %3C612::AID – CNCR2820540403 %3E3.0. CO;2-B/-abstract.
170
H. Gilbert Welch and William C. Black, Using autopsy series to estimate the disease “reservoir” for ductal carcinoma in situ of the breast: how much more breast cancer can we find? Annals of Internal Medicine, vol. 127, no. 11, Dec. 1997, pp. 1023–1028, www.vaoutcomes.org/papers/Autopsy_Series.pdf.
171
Точная статистика зависит от страны и демографической ситуации – а также от того, насколько активно занимаются онкоскринингом молочных желез в стране, – поэтому такие данные получить трудно. Полный обзор британской статистики см. здесь: https://www.cancerresearchuk.org/health-professional/cancer-statistics/statistics-by-cancer-type/breast-cancer.
172
Из личной беседы с Джонатаном Каневским.
173
Breakthrough method predicts risk of DCIS becoming invasive breast cancer, Artemis, May 2010, http://hopkinsbreastcenter.org/artemis/201005/3.html.
174
H. Gilbert Welch, Philip C. Prorok, A. James O’Malley and Barnett S. Kramer, Breast-cancer tumor size, overdiagnosis, and mammography screening effectiveness, New England Journal of Medicine, vol. 375, 2016, pp. 1438–1447, http://nejm.org/doi/full/10.1056/NEJMoa1600249.
175
Independent UK Panel on Breast Cancer Screening, The benefits and harms of breast cancer screening: an independent review, Lancet, vol. 380, no. 9855, 30 Oct. 2012, pp. 1778–1786, http://thelancet.com/journals/lancet/article/PIIS0140–6736 (12) 61611–0/abstract.
176
Из личной беседы.
177
Andrew H. Beck, Ankur R. Sangoi, Samuel Leung, Robert J. Marinelli, Torsten O. Nielsen, Marc J. van de Vijver, Robert B. West, Matt van de Rijn and Daphne Koller, Systematic analysis of breast cancer morphology uncovers stromal features associated with survival, Science Transitional Medicine, 19 Dec. 2014, https://becklab.hms.harvard.edu/files/becklab/files/sci_transl_med-2011-beck-108ra113.pdf.
178
Phi Vu Tran, A fully convolutional neural network for cardiac segmentation in short-axis MRI, 27 April 2017, https://arxiv.org/pdf/1604.00494.pdf.
179
Emphysema, Imaging Analytics, Zebra Medical, https://zebra-med.com/algorithms/lungs/.